You cannot read all of the news, every day. There is simply too much information for even a dedicated and specialized observer to consume it all, so someone or something has to make choices. Traditionally, we rely on some other person to tell us what to see: the editor of a newspaper decides what goes on the front page, the reviewer tells us what movies are worth it. Recently, we have been able to distribute this mediation process across wider communities: sites like Digg, StumbleUpon, or Slashdot all represent the collective opinions of thousands of people.
The next step is intelligent news agents. Google (search, news, reader, etc.) can already be configured to deliver to us only that information we think we might want to see. It’s not hard to imagine much more sophisticated agents that would scour the internet for items of interest.
In today’s context, it’s easy to see how such agents could actually be implemented. Sophisitacted customer preference engines are already capable of telling us what products we might like to consume — the best example is Amazon’s recommendation engine. It’s not a big leap to imagine using the same sort of algorithms to model the kinds of blog articles, web pages, youtube videos, etc. that we might enjoy consuming, and then deliver these things to us.
There is a serious problem with this. You’re going to get exactly what you ask for, and only that.
True, we all do this already. We read books and consume media which more or less confirm our existing opinions. This effect is visible as clustering in what we consume, as in this example of Amazon sales data for political books in 2008.
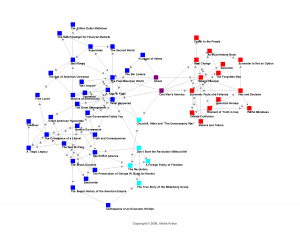
This image is from a beautiful analysis by orgnet.com. Basically, people buy either the red books or the blue books, but usually not both. The same sorts of patterns hold for movies, blogs, newspapers, ideologies, religions, and human beliefs of all kinds. This is a problem; but at least you can usually see the other color of books when you walk into Borders. If we end up relying on trainable agents for all of our information, we risk completely blacking out anything that disagrees with what we already believe.
I propose a simple solution. Automatic network analyses like the one above — of books, or articles, or web pages — could easily pinpoint the information sources that would expose me to the maximum novelty in the minimum time. If my goal is to gain a deep understanding of the entire scope of human discourse, rather than just the parts of it I already agree with, then it would be very simple to program my agent to bring to me exactly those things that would most rapidly give me insight into those regions of information space which are most vital and least known to me. I imagine some metric like “highest degree node most distant from the nodes I’ve already visited” would would work handily.
You can infer a lot about somewhat from the information they currently consume. If my agent noticed that I was a liberal, it could make me understand the conservative world-view, and vice-versa. If my agent detected that I was ignorant of certain crucial aspects of Chinese culture and politics, it could reccomend a primer article. Or it might deduce that I needed to understand just slightly more physics to participate meaningfully in the climate change debate, or decide (based on my movie viewing habits) that it was high time I review the influential films of Orson Welles. Of course, I might in turn decide that I actually, truly, don’t care about film at all; but the very act of excluding specific subjects or categories of thought would force us, consciously, to admit to the boundaries of our mental worlds.
We could program our information gathering systems to challenge us, concisely and effectively, if we so want. Intelligent agents could be mere sycophants, or they could be teachers.